Multimodal Image Fusion Using Conditional Diffusion Models: A Revolutionary Approach for Better Image Clarity
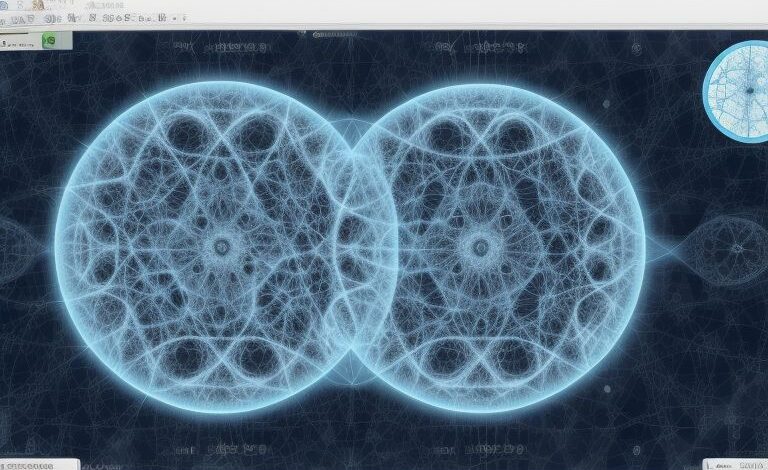
Multimodal Image Fusion Using Conditional Diffusion Models: A Revolutionary Approach for Better Image Clarity Image fusion through conditional diffusion models brings revolutionary possibilities for optimizing visual clarity across multiple sources in a single combined picture due to its advanced methodology.
The use of conditional diffusion models in multimodal image fusion methods changes our current methods of combining images from different sources. This advanced technique enables the combination of images into merged results which become more detailed and precise in their understanding. The approach proves exceptionally useful in medical imaging for accurate diagnosis because all details remain crucial.
The technology of multimodal image fusion through conditional diffusion models functions by unifying multiple images into improved visuals which maintain all necessary details. The technology serves applications well where we need to view major pictures along with fine details within one clear image such as infrared-visible image fusion and medical imaging.
Table of Contents
The process of combining image-related data presents a technique named Multimodal Image Fusion Using Conditional Diffusion Models.
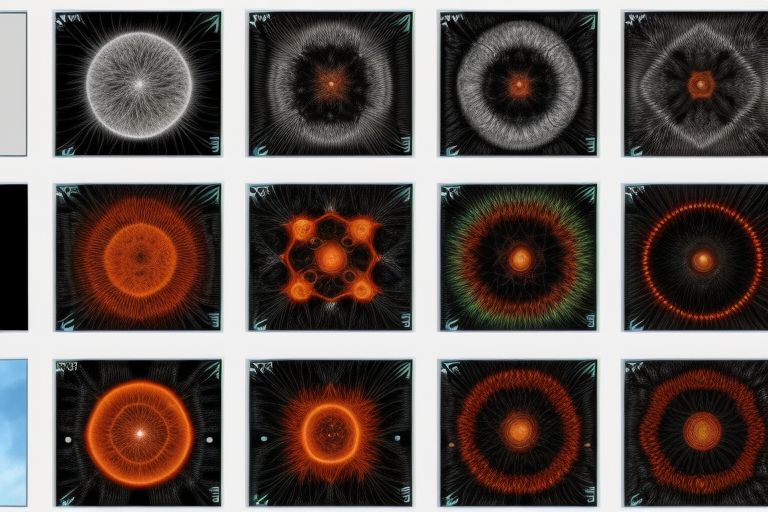
Multimodal Image Fusion Using Conditional Diffusion Models Conditional diffusion models create a fresh method through which different image sources can merge their content to create enhanced visibility in the output. You possess images of the same item which one was obtained through infrared camera technology and the second was acquired through standard visible light camera technology. The method allows you to combine the two images into one unified picture. The objective here is to extract helpful elements from both images to generate a single result that provides more detail while remaining easy to comprehend.
The technique requires a particular model structure named “diffusion model” for its operation. The procedure smooths image content transitions while maintaining all critical elements present in both sources. The diffusion model directs the image combination process as a guide. During fusion the model safeguards the major features including shapes and textures which originate from separate images. The use of conditional diffusion models for multimodal image fusion proves beneficial throughout various fields such as medical imaging and security.
Why Conditional Diffusion Models Are the Future of Image Fusion
Conditional diffusion models have become the leading force in image fusion since they produce superior results compared to traditional methods. The main limitation of conventional fusion approaches involves their unstable operation which results in poor outcome quality. GANs and other older models sometimes fail to create images with detailed contents and produce unwanted deviations while losing critical elements within the pictures. The outcomes generated by these methods have lower reliability because of the irregularities present in the results.
Conditional diffusion models solve the present problems which arise in multimodal image fusion systems. The main distinction of these models relies on their method to combine images through controlled processes. These models direct their fusion process with data obtained from the original images to produce final outputs instead of engaging in random speculation. The process results in pictures that maintain both strength and reliability. The medical field depends heavily on precise images so these models have become accepted sources because of their dependable output.
How They Work in Simple Terms
Users can picture multimodal image fusion that uses conditional diffusion models as a painting operation. Two different images function as paints which need combination to produce a mixed and altered result. A painting artist provides the same function as the diffusion model which selects proper paint amounts for perfect blending. The artist can combine data from these two paint types to generate an excellent final artwork that includes all desirable characteristics.
How Multimodal Image Fusion Improves Clarity in Medical Imaging
Medical imaging professionals need complete visibility of full-sized objects together with detailed information for correct diagnosis. Doctors who perform MRI or CT scans receive important structural data however they often miss functional details. Conditioned diffusion models for multimodal image fusion permit medical professionals to unite functional and structural information as a single distinct medical image. Such combination makes doctors more effective at identifying rare medical conditions that earlier detection systems failed to detect.
During fusion processing both major structural characteristics and minute elements such as blood vessels and tiny fractures are maintained. The combined image enables a comprehensive representation of the actual state. Conditional diffusion models enhance medical image clarity which enables doctors to reach improved diagnosis decisions.
The Importance of Combining Different Image Types

Various imaging scans exist which medical practitioners use to obtain multi-dimensional patient health information. A patient undergoing an MRI scan will receive clear images of the brain structures yet a PET scan reveals which brain regions are currently active. The combination of two different brain image types through conditional diffusion models allows doctors to view structural and activity information as one detailed image. Research on diseases such as cancer requires complete information about tumor shape and its behavioral characteristics to develop an accurate understanding of conditions.
Benefits of Using Conditional Diffusion Models in Multimodal Image Fusion
The key benefit from implementing multimodal image fusion through conditional diffusion models is its ability to operate with flexible methods. The new models show superior flexibility because they can easily adapt to processing multiple varieties of data when compared to previous methods. The adaptation capability of diffusion models extends to all types of infrared images and medical scans and additional image sources. The models prove more suitable for practical uses because they can process images acquired from different types of sensor systems.
The benefit behind fused images from this method includes top-tier image quality. The conditional diffusion model achieves superior image blending outcomes because it maintains thorough control over the processing steps. The process needs particular focus when merging images because high precision requirements exist in surveillance or medical diagnosis scenarios. The analysis of fostered images becomes easier because they present better clarity while keeping essential details intact.
Efficiency and Accuracy
The efficiency of conditional diffusion models in multimodal image fusion decreases the time for image analysis and processing activities. The models perform fast processing of data from multiple sources which results in correct analysis. Quick decision-making processes in security applications depend heavily on analyzing images according to their demands.
Applications of Multimodal Image Fusion in Real-World Scenarios
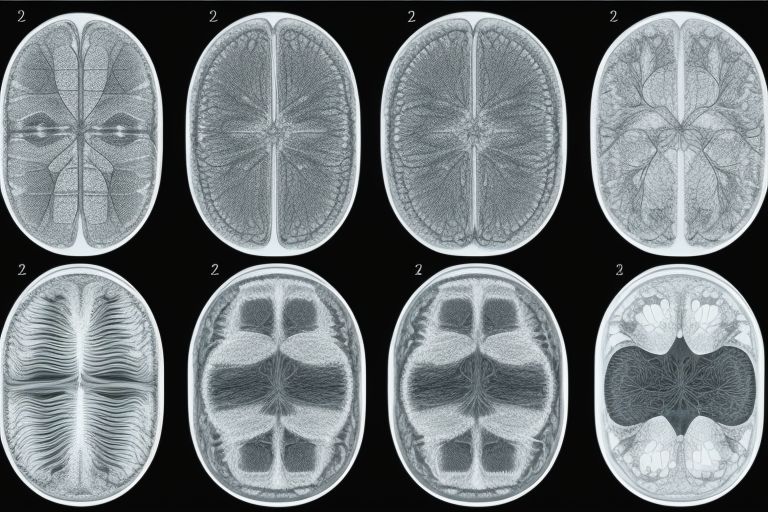
The implementation of conditional diffusion models for multimodal image fusion benefits numerous modern applications that cover security and healthcare systems. Healthcare doctors rely on image fusion to gain improved visualization of patients’ medical circumstances. The models contribute to generating expanded security images by integrating visual elements acquired from different camera systems to monitor particular areas. These new models provide professionals with improved ways to make decisions as they process detailed and clear images.
Different sensors provide various environmental data to autonomous vehicles through this method. The application of fusion models by these vehicles generates an enhanced overview of road conditions which promotes improved driving safety together with superior operational output. Conditional diffusion models used for multimodal image fusion will establish a vital position in upcoming technological innovations as application numbers increase.
Real-World Examples
Healthcare professionals utilize conditional diffusion models in multimodal image fusion technology to identify cancer along with heart diseases during medical diagnosis. PET image combination with CT scans provides doctors with simultaneous visualization of tumor position while monitoring its activity. Security surveillance systems become more efficient at threat detection during low-light situations when infrared and visible light images are combined for analysis. The demonstrated applications demonstrate that this technology provides both strength and diversity in its applications.
Conclusion
Multimodal image fusion through conditional diffusion models operates as a strong and beneficial technique that unites distinct image types into clearer and more purposeful final outputs. This technique generates superior outcomes that serve medical applications and defense systems as well as automotive automation. The models merge choice elements found across pictures to reveal extra details which enable users to make informed judgment. The technology enhances daily image usage by providing benefits to doctor patient diagnosis and car autonomous driving operations.
The method stands out through its fundamental capability to merge different data sources which boosts multiple business sectors. By uniting various technology capabilities the system delivers precise images that aid problem-solving procedures with improved efficiency. Our development of new multimodal image fusion methods with conditional diffusion models will advance numerous life aspects through upcoming exciting innovations.
FAQs
The method of combining images through different sources constitutes multimodal image fusion.
Image fusion based on multiple sources combines pictures to generate clearer detailed images to help users solve different problems.
Conditional diffusion models utilize a guidance mechanism to achieve superior results in image fusion tasks.
By implementing conditional diffusion models into image blending applications the fusion process gains better accuracy for maintaining critical image details in resulting images.
The technology has potential applications in healthcare organizations.
This technology permits medical facilities to unite different imaging solutions namely CT scans with MRIs for enhanced diagnostics and therapeutic approaches.
Multimodal image fusion serves medical applications exclusively.
This technology exists for multiple practical applications such as security operations and autonomous vehicle development and environmental monitoring purposes.
The major benefits of diffusion models make image fusion possible.
The fusion process performed by diffusion models creates better and less unpredictable results through precise image integration to yield.generate high-quality outputs.